How Does AI Process Data: From Bytes to Brilliance
AI has revolutionized how we process data, enabling machines to analyze and interpret vast amounts of information quickly and efficiently. In this comprehensive guide, we'll explore how AI processes data, understand the importance of quality data, and delve into the challenges it faces.
Understanding how AI data processing works
To effectively harness the power of AI, it’s essential to understand and implement the key steps in data processing:
- Data collection from multiple sources. Gather relevant information from databases, the web, and user interactions.
- Data cleaning and preparation. Filter out irrelevant information, correct errors, and organize data into a structured format.
- Application of machine learning algorithms. Analyze data to identify patterns and make predictions.
- Utilization of deep learning neural networks. Use artificial neural networks for high-accuracy processing, especially in complex tasks.
Data collection: casting the net
The first step in the data processing journey is collecting data. This involves gathering relevant information from various sources, such as databases, websites, and user interactions. You can imagine data collection as casting a wide net into the digital ocean, capturing bits and bytes of information that hold the potential to unveil valuable insights.
Data cleaning and preparation: getting ready for analysis
Once the data is collected, it needs to be prepared for analysis. This step is crucial as raw data often contains noise, inconsistencies, and errors. Data cleaning and preparation involve:
- filtering out irrelevant information;
- correcting errors;
- organizing the data into a structured format.
Think of it as sifting through the catch from your net, discarding the debris, and keeping only the valuable pieces.
Application of machine learning algorithms: finding patterns
With clean and prepared data, the next step is to apply machine learning algorithms. These algorithms analyze the data, identifying patterns and making predictions without explicit programming. They learn from the data and improve over time, becoming more accurate and efficient. This process is like teaching a digital assistant to recognize patterns and make informed decisions based on historical data.
Utilization of deep learning neural networks: enhancing precision
Deep learning, a subset of machine learning, takes data processing further. It uses artificial neural networks to manage vast amounts of data with exceptional accuracy. These networks mimic the human brain, making them particularly effective in complex tasks like image and speech recognition. Deep learning continuously refines its models, enhancing precision and delivering impressive results.
Different AI techniques for data processing
AI encompasses various techniques for processing data. Let's explore two prominent techniques: machine learning and deep learning.
Machine learning and data processing
Machine learning algorithms serve as the backbone of numerous applications across various industries. These algorithms can swiftly recognize patterns and generate accurate predictions by processing vast amounts of data. From image recognition in healthcare to fraud detection in finance, the versatility of machine learning in data processing is truly remarkable.
Moreover, the iterative nature of machine learning allows algorithms to continuously improve their performance over time, making them invaluable assets for organizations seeking to extract meaningful insights from complex datasets.
Deep learning and data processing
Deep learning, a subfield of machine learning, takes data processing to new heights by focusing on training artificial neural networks. These networks, inspired by the intricate structure and functionality of the human brain, possess the capability to process massive volumes of data with unparalleled efficiency.
One of the standout features of deep learning algorithms is their exceptional performance in tasks such as image and speech recognition. By harnessing the power of neural networks, deep learning has spearheaded groundbreaking advancements in fields like computer vision and natural language processing.
This technological innovation has empowered machines to comprehend and generate human-like content, paving the way for a future where AI seamlessly integrates with our daily lives.
The importance of quality data in AI processing
Data quality is of utmost importance in AI processing. The accuracy and reliability of AI systems heavily rely on the quality of the data they receive.
High-quality data is the foundation for AI algorithms to generate meaningful insights and make informed decisions. Without reliable data, AI systems may produce misleading results, impacting various sectors such as:
- healthcare;
- finance;
- transportation.
Impact of inaccurate data
Inaccurate data can lead to flawed predictions and decisions. The AI system might learn incorrect patterns if the input data contains errors or biases, leading to inaccurate outputs.
Furthermore, the consequences of relying on inaccurate data extend beyond individual predictions. In sectors like autonomous driving, a single error in data input could have catastrophic implications, highlighting the critical need for data accuracy in AI systems.
Moreover, inaccurate data can have far-reaching consequences. In domains like healthcare or finance, inaccurate predictions can potentially harm individuals or businesses. Therefore, ensuring data accuracy is crucial for reliable AI processing.
Ensuring data quality
Data transparency and audibility are essential factors in ensuring data quality. Organizations should maintain proper documentation and traceability of the data used in AI processing.
Implementing data quality frameworks and regular data quality assessments can help organizations identify and rectify any issues in the data pipeline, ensuring that AI systems operate with the highest accuracy and reliability.
Challenges in AI data processing
Despite the remarkable progress in AI, there are still challenges in data processing that need to be addressed.
Data privacy and security concerns
As AI relies heavily on data, privacy and security concerns arise. Organizations need to ensure that data collected and used for AI purposes comply with relevant regulations.
Overcoming data bias
Data bias occurs when training data doesn't represent real-world facts and accepted opinions. As a result, AI systems may exhibit biased behavior, perpetuating prejudices and stereotypes.
To address data bias, organizations need to carefully curate their training datasets and test for biases. Diversity in the data collection process and AI model development can help mitigate bias.
Furthermore, ongoing monitoring and evaluation of AI systems can help identify and rectify any biases that may emerge over time. This iterative approach ensures continuous improvement and fairness in AI data processing.
Unlock the full potential of AI with quality data
AI data processing transforms vast amounts of raw information into actionable insights through a series of essential steps. The effectiveness of these processes hinges on the quality and accuracy of the data used, underscoring the importance of robust data governance and addressing challenges such as data privacy, security, and bias.
By leveraging high-quality data and continuously refining AI models, organizations can unlock the full potential of AI, driving innovation and making informed decisions across various sectors.
If you're looking for the best tools for the job, why not check out web scrapers that help you collect data and improve AI performance? They're perfect for collecting large amounts of data from the web without ever running into issues.
About the author
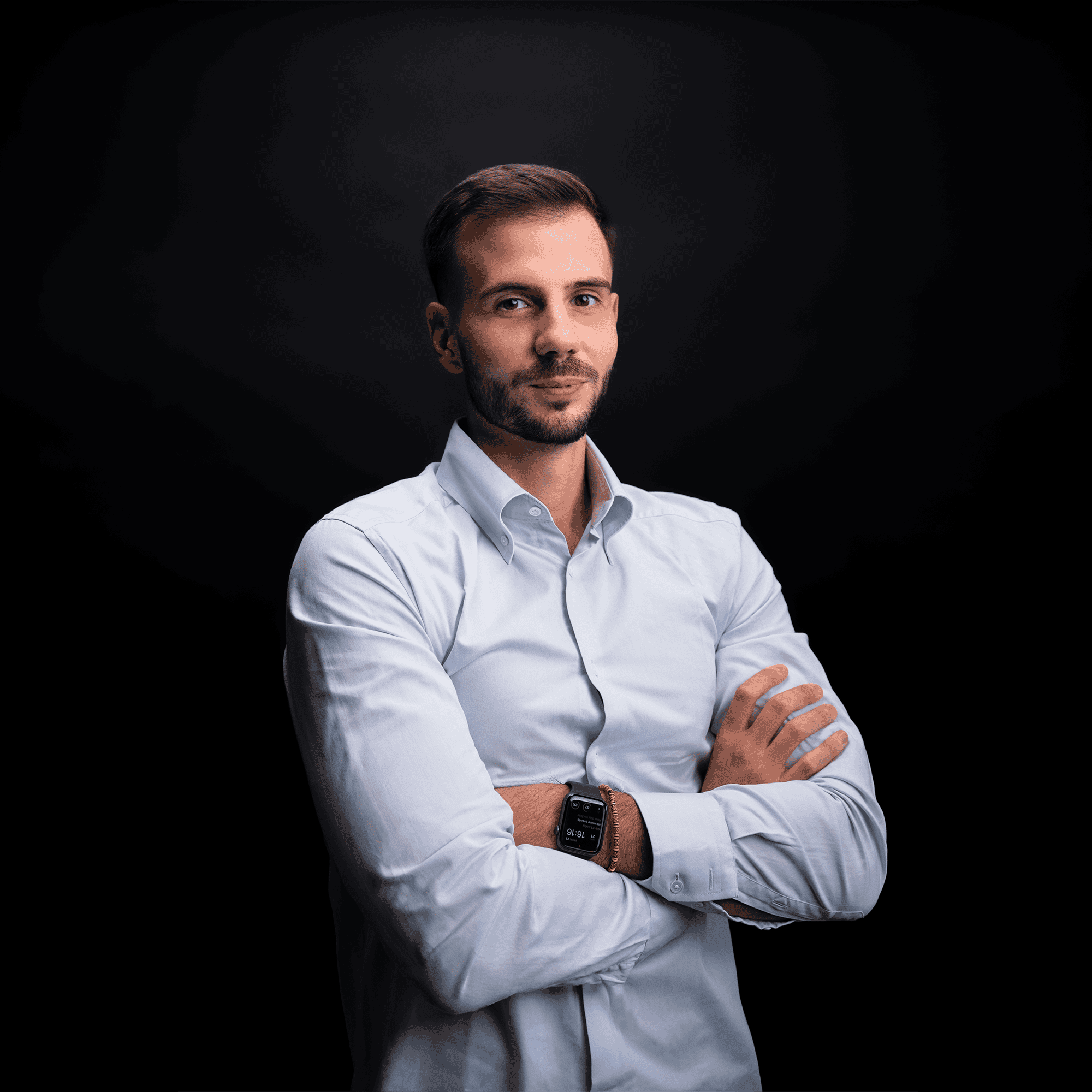
Martin Ganchev
VP Enterprise Partnerships
Martin, aka the driving force behind our business expansion, is extremely passionate about exploring fresh opportunities, fostering lasting relationships in the proxy market, and, of course, sharing his insights with you.
All information on Smartproxy Blog is provided on an as is basis and for informational purposes only. We make no representation and disclaim all liability with respect to your use of any information contained on Smartproxy Blog or any third-party websites that may belinked therein.